🌍 All
About us
Digitalization
News
Startups
Development
Design
How AI-Powered Testing Tools are Revolutionising QA/QC
Marek Pałys
Dec 03, 2024・7 min read
Table of Content
Understanding AI in QA/QC
Key Benefits of AI Testing Tools
Popular AI Testing Tools
Challenges and Considerations
Future of AI in QA/QC
The advent of AI-powered testing tools has marked a significant shift in how industries maintain standards and ensure product reliability. These innovative tools are reshaping traditional testing processes by introducing automation, precision, and efficiency, thus driving improvements in both speed and accuracy. By harnessing the power of artificial intelligence, organisations can now perform comprehensive assessments of test scripts that were once time-consuming and prone to human error. This transformation not only enhances the quality of products but also optimises resources, making it a practical solution for businesses of all sizes. As we delve deeper into this topic, we will explore the myriad ways AI is revolutionising QA/QC, offering insights into the future of quality management.
Understanding AI in QA/QC
What is AI-Powered Testing?
AI-powered testing refers to the use of artificial intelligence technologies in executing quality assurance and quality control tasks. Traditional testing methods often rely on manual procedures, which can be time-consuming and susceptible to human error. In contrast to manual testing, AI-powered testing employs algorithms and machine learning models to automate these processes. These tools can simulate user interactions, predict potential faults, and analyse data at a scale far beyond human capability. By doing so, they provide faster feedback and more accurate results. AI-driven testing tools can adapt to new data, learn from previous tests, and improve over time. This adaptability makes them incredibly effective in maintaining high standards of product quality and reliability. As a result, businesses can reduce costs, increase efficiency, and ensure their products meet the ever-evolving industry standards. AI-powered testing is a game-changer in the field of QA/QC, offering unprecedented capabilities for comprehensive quality management.
The Role of AI in Quality Assurance
AI plays a pivotal role in transforming quality assurance processes by enhancing the accuracy and speed of testing methods. One key aspect is its ability to automate repetitive tasks, such as data entry and regression testing. This automation reduces the likelihood of human error and frees up QA professionals to focus on more complex issues. Moreover, AI can analyse vast amounts of data to detect patterns and anomalies that might indicate potential defects. This capability allows for proactive identification and resolution of problems before they escalate. AI also facilitates predictive analysis, helping organisations foresee potential failures and implement preventative measures. By using machine learning algorithms, AI systems can continuously learn and adapt, improving their performance testing effectiveness over time. This leads to more reliable and efficient QA processes, ensuring higher quality products and services. In essence, AI not only optimises current QA practices but also paves the way for future innovations in quality management.
How AI Improves Quality Control
AI enhances quality control by providing precise, real-time insights into production processes. One of the main advantages is its ability to continuously monitor and analyse production data, detecting deviations from established standards with high accuracy. This capability enables immediate corrective actions, ensuring consistent product quality. AI systems and test automation can also perform visual inspections using image recognition technology, identifying defects that might be missed by the human eye. This leads to higher precision in detecting flaws and reduces the risk of defective products reaching consumers. Furthermore, AI's predictive analytics can forecast potential production issues, allowing companies to address them proactively. By integrating AI into quality control, manufacturers can optimise their processes, reduce waste, and improve efficiency. This results in cost savings and enhanced product reliability. Overall, AI transforms quality control from a reactive to a proactive discipline, significantly boosting the ability to maintain high standards and meet customer expectations.
Key Benefits of AI Testing Tools
Enhancing Efficiency and Accuracy
AI testing tools significantly boost efficiency and accuracy within quality assurance and quality control frameworks. Automation stands at the core of this enhancement, as AI can execute repetitive and complex testing tasks much faster than humans. This speed allows QA teams to run more tests in a shorter period, reducing the time to market for new products. Furthermore, AI’s precision minimises the risk of oversight during the testing process, ensuring that even the smallest defects are identified and addressed. These tools can also learn from historical data, continuously improving their testing strategies and outcomes. As a result, businesses experience fewer false positives and negatives, leading to more reliable test results. Additionally, the ability of AI to handle vast datasets enables comprehensive testing without the resource strain typical in manual processes. By integrating AI testing tools, organisations can achieve higher standards of quality while optimising their operational resources, ultimately enhancing overall productivity and customer satisfaction.
Reducing Human Error
AI testing tools play a crucial role in minimising human error in quality assurance and quality control processes. Human error, often stemming from fatigue or oversight, can lead to significant flaws in products or services. AI addresses this challenge by automating routine and complex testing tasks, which reduces the dependency on manual intervention. This automation ensures consistent execution of tests, eliminating the variability that human testers might introduce into test execution. Additionally, AI systems can handle large volumes of data and conduct thorough analyses that would be impractical for human testers to perform accurately and efficiently. By relying on algorithms and machine learning, AI testing tools offer precise and repeatable results, reducing the likelihood of errors slipping through the cracks. The reduction of human error not only improves the reliability and quality of outputs but also builds greater trust among consumers. In essence, AI testing tools provide a more robust framework for achieving high-quality standards consistently.
Cost-Effectiveness and Scalability
AI testing tools offer significant advantages in terms of cost-effectiveness and scalability, making them an attractive option for businesses aiming to optimise their QA/QC processes. By automating routine testing tasks, AI reduces the need for extensive human resources, cutting down on labour costs. This efficiency allows companies to allocate their workforce to more strategic roles, enhancing overall productivity. Additionally, AI tools can operate around the clock without fatigue, further maximising resource utilisation. Scalability is another major benefit of continuous testing alone, as AI systems can easily adapt to increased testing demands. Whether a company is launching a new product line or expanding into new markets, AI testing tools can handle the additional workload without a proportional increase in costs. This flexibility is particularly beneficial for startups and growing businesses, as it provides the ability to scale operations efficiently. By integrating AI testing tools, organisations can achieve cost savings while maintaining robust quality standards, ensuring long-term growth and competitiveness.
Popular AI Testing Tools
Leading Software in the Market
Several AI-powered testing tools have emerged as leaders in the QA/QC market, each offering unique features that cater to different testing needs. One prominent tool is Testim, known for its advanced AI capabilities that simplify the process of creating and executing automated tests. It is test automation platform utilises machine learning to adapt to changes in the application being tested, reducing maintenance efforts. Another key player is Applitools, which specialises in visual testing and monitoring, providing robust solutions for ensuring UI consistency across different devices and browsers. Additionally, Functionize combines machine learning with cloud computing to deliver highly scalable testing solutions, allowing teams to run complex tests in parallel. These tools not only enhance testing accuracy but also streamline workflows, making them indispensable assets for modern QA teams. By leveraging these leading software solutions, companies can achieve superior testing outcomes, ensuring their products meet the highest quality standards while staying competitive in fast-paced markets.
Features to Look For
When evaluating AI testing tools, it's important to consider features that align with your specific quality assurance needs. Automation capabilities are paramount; look for tools that can seamlessly automate repetitive tasks and adapt to changes in the testing environment. Machine learning integration is another key feature of test automation tool, as it allows the tool to improve over time, reducing maintenance efforts. Additionally, robust data analysis capabilities are essential for identifying patterns and anomalies quickly. Ensure the tool offers comprehensive reporting features, providing clear insights and actionable recommendations for improving product quality. Compatibility with various platforms and environments can also enhance the tool's versatility, allowing it to be integrated into existing workflows without hassle. Lastly, consider user-friendliness and support services; a tool that's intuitive and backed by reliable customer support can significantly ease the transition and maximise the tool's potential. By prioritising these features, organisations can select AI testing tools that drive efficiency and ensure high standards.
Case Studies of Successful Implementation
Numerous organisations have successfully implemented AI testing tools to enhance their QA/QC processes, yielding impressive results. For instance, a leading e-commerce platform integrated AI-powered testing to streamline its release cycles. By automating repetitive tests and leveraging machine learning for predictive analytics, the company reduced testing time by 30% and improved defect detection rates. Another example is a financial services firm that adopted AI tools to test maintenance and ensure compliance with regulatory standards. The AI system's ability to process large datasets allowed for more comprehensive testing, resulting in fewer compliance issues and a 20% reduction in operational costs. A tech startup focusing on mobile applications used AI testing to maintain consistent user experiences across devices. The tool's visual testing capabilities ensured UI consistency, contributing to a significant increase in user satisfaction. These case studies demonstrate the tangible benefits of AI testing tools, showcasing their potential to transform quality assurance practices across diverse industries.
Challenges and Considerations
Overcoming Integration Hurdles
Integrating AI testing tools into existing QA/QC workflows can pose several challenges, but these can be managed with strategic planning and implementation. One common hurdle is the compatibility of AI tools with legacy systems. Addressing this requires a thorough assessment of current systems and potential tool adjustments to ensure seamless integration of software testing itself. Training staff to effectively use these new tools is another critical step. Providing comprehensive training programmes can help employees adapt to AI technologies, ensuring a smoother transition. Additionally, data management is crucial, as AI tools rely heavily on data for accurate testing and analysis. Establishing robust data governance practices can help manage this aspect efficiently. Collaboration between IT and QA teams is essential to address technical and operational challenges swiftly. By tackling these integration hurdles methodically, organisations can fully leverage the benefits of AI testing tools, enhancing their quality assurance processes while minimising disruptions.
Addressing Data Privacy Concerns
Data privacy is a significant concern when deploying AI testing tools, as these systems often require access to sensitive data for effective analysis. To address these concerns, organisations should implement robust data protection protocols. This includes encrypting data both in transit and at rest, ensuring that only authorised personnel have access to critical information. Adopting privacy-by-design principles during test creation can also help integrate privacy considerations into the system architecture from the outset. Additionally, it is crucial to comply with relevant data protection regulations, such as the General Data Protection Regulation (GDPR) in Europe, to avoid legal complications. Regular audits and assessments can ensure ongoing compliance and identify potential vulnerabilities. Providing transparency about data usage and obtaining explicit consent from users can further mitigate privacy risks. By prioritising data privacy, companies can leverage AI testing tools effectively while maintaining customer trust and safeguarding sensitive information.
Balancing Automation and Human Oversight
Striking the right balance between automation and human oversight is crucial in the effective implementation of AI testing tools. While automation offers efficiency and accuracy, it cannot completely replace human intuition and expertise. Human oversight is essential for interpreting nuanced test results and making strategic decisions that machines might not handle contextually. To achieve this balance, organisations should define clear roles for AI tools and human testers. AI can handle repetitive and data-intensive tasks, while human testers focus on exploratory testing and decision-making based on complex scenarios. Regular reviews and updates to testing strategies are necessary to adapt to changing requirements and technological advancements. Furthermore, fostering collaboration between AI systems and human teams can enhance understanding and integration. By maintaining this synergy, businesses can maximise the benefits of AI, ensuring that automated testing processes complement human insights, leading to more robust and reliable quality assurance practices.
Future of AI in QA/QC
Emerging Trends and Technologies
The future of AI in QA/QC is set to be shaped by several emerging trends and technologies that promise to further enhance testing processes. One significant trend is the increasing integration of AI with Internet of Things (IoT) devices, enabling real-time monitoring and analysis of product performance in various environments. This convergence allows for more comprehensive quality assessments and quicker identification of potential issues. Another development is the rise of explainable AI, which seeks to make AI decision-making processes more transparent. This is crucial for gaining trust in AI-driven testing results and ensuring regulatory compliance. Additionally, advancements in natural language processing are enabling more intuitive test case creation and management, reducing complexity for users. The use of blockchain for maintaining secure and immutable records of all test cases and data is also gaining traction. These technologies, combined, will drive a new era of precision, efficiency, and reliability in QA/QC, transforming how organisations approach quality management.
Potential Impact on Industries
The integration of AI in QA/QC is poised to significantly impact various industries, enhancing productivity and product quality across the board. In manufacturing, AI can optimise production lines by predicting equipment failures and ensuring consistent quality output, reducing downtime and waste. The healthcare sector stands to benefit from AI's ability to process large datasets swiftly, improving the accuracy and reliability of diagnostic tools. In the software industry, AI-driven testing can expedite development cycles, ensuring robust applications with fewer bugs. The automotive industry will also see improvements, particularly in the development load testing of autonomous vehicles, where rigorous testing is crucial for safety. Additionally, consumer goods companies can leverage AI to monitor supply chains, ensuring that products meet quality standards from production to distribution. Across all these sectors, AI in QA/QC can lead to cost savings, better compliance with regulations, and enhanced customer satisfaction, ultimately driving growth and innovation.
Preparing for Continuous Advancements
As AI technologies continue to evolve, organisations must prepare to adapt their QA/QC strategies to leverage these advancements effectively. Staying informed about the latest developments in AI is crucial, enabling companies to identify opportunities for integrating new tools and methodologies into their quality assurance processes. Investing in ongoing training and development for QA teams ensures that they remain proficient in using AI tools, fostering a culture of continuous learning. Additionally, establishing flexible infrastructures can facilitate the incorporation of emerging AI technologies without significant disruptions. Collaborating with AI solution providers can offer insights into best practices and innovative applications software testing tools, helping businesses remain competitive. Moreover, fostering a mindset that embraces change and innovation can drive successful adaptation. By being proactive and strategic, organisations can not only keep pace with AI advancements but also harness them to enhance quality management, leading to sustained improvements in product quality and customer satisfaction.
FAQ
What are AI-powered testing tools?
AI-powered testing tools use artificial intelligence to automate and enhance QA/QC processes, improving efficiency, accuracy, and consistency in detecting and resolving defects.
How do AI testing tools work?
These tools leverage machine learning algorithms and data analysis to execute test scripts, simulate user interactions, and identify patterns or anomalies for faster and more accurate testing.
What are the benefits of AI testing tools?
Key benefits include enhanced efficiency, reduced human error, cost savings, scalability, and the ability to handle complex testing scenarios across diverse environments.
How do AI tools improve QA/QC?
AI tools automate repetitive tasks, provide predictive analytics for early defect detection, and perform real-time monitoring to ensure high-quality standards are maintained.
What features should I look for in AI testing tools?
Look for automation capabilities, machine learning integration, data analysis features, comprehensive reporting, platform compatibility, and user-friendly interfaces.
What industries benefit most from AI testing tools?
Industries such as software development, manufacturing, healthcare, automotive, and finance see significant benefits, including improved efficiency, compliance, and product quality.
Can AI testing tools replace manual testing?
No, AI testing tools complement manual testing. While they automate routine tasks, human oversight remains essential for exploratory testing and assessing user experience.
What are some popular AI testing tools?
Leading tools include Testim for automated test creation, Applitools for visual testing, and Functionize for scalable cloud-based testing solutions.
How do AI tools enhance visual testing?
AI-powered visual testing tools use image recognition technology to identify UI inconsistencies across devices and browsers, ensuring a seamless user experience.
What challenges come with implementing AI testing tools?
Challenges include integrating AI tools with legacy systems, ensuring data privacy, providing adequate training, and balancing automation with human oversight.
How does AI address data privacy in testing?
AI tools can incorporate encryption, data anonymization, and compliance with regulations like GDPR to protect sensitive information during testing.
What is the future of AI in QA/QC?
The future includes integration with IoT for real-time monitoring, explainable AI for transparency, blockchain for secure test records, and advancements in natural language processing for test creation.
How do AI testing tools impact cost-effectiveness?
AI testing tools reduce costs by automating repetitive tasks, minimizing human error, and scaling efficiently to handle increased testing demands without proportional cost increases.
What is the role of predictive analytics in AI testing tools?
Predictive analytics identify potential issues before they occur, enabling proactive measures to maintain quality and reduce downtime in production processes.
How can businesses prepare for AI advancements in QA/QC?
Businesses should invest in staff training, adopt flexible infrastructures, collaborate with AI solution providers, and stay informed about emerging trends and technologies.
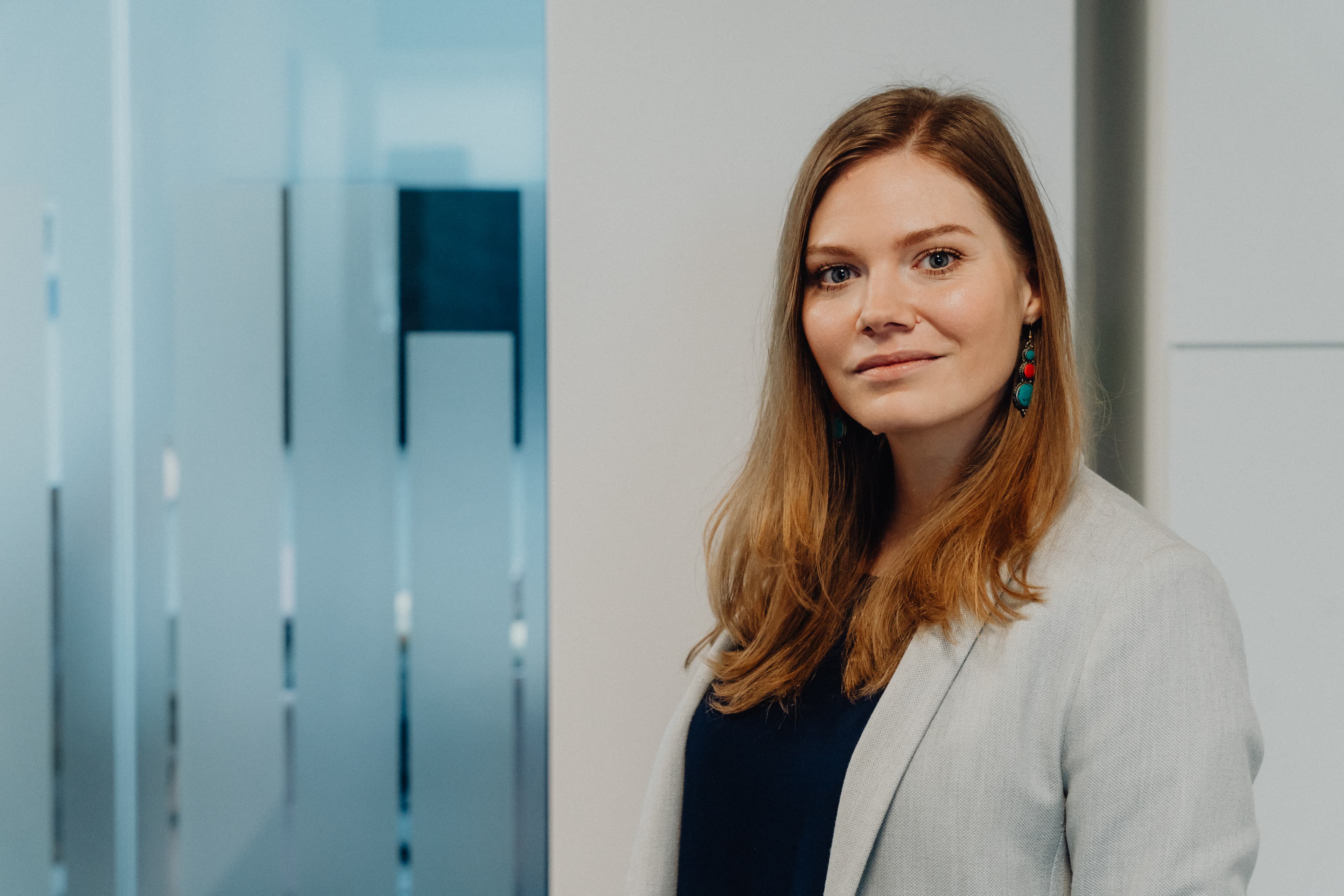
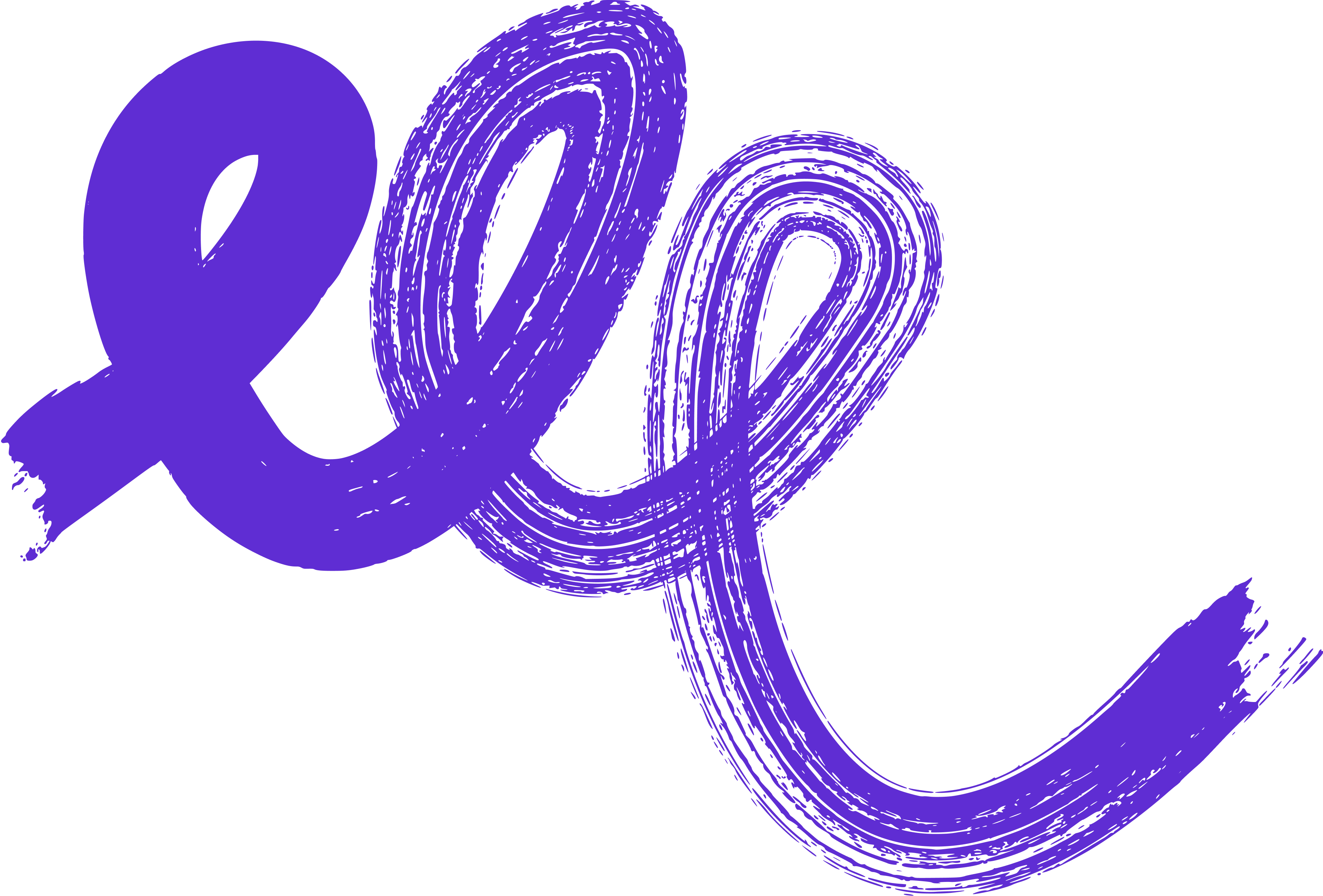
You may also
like...
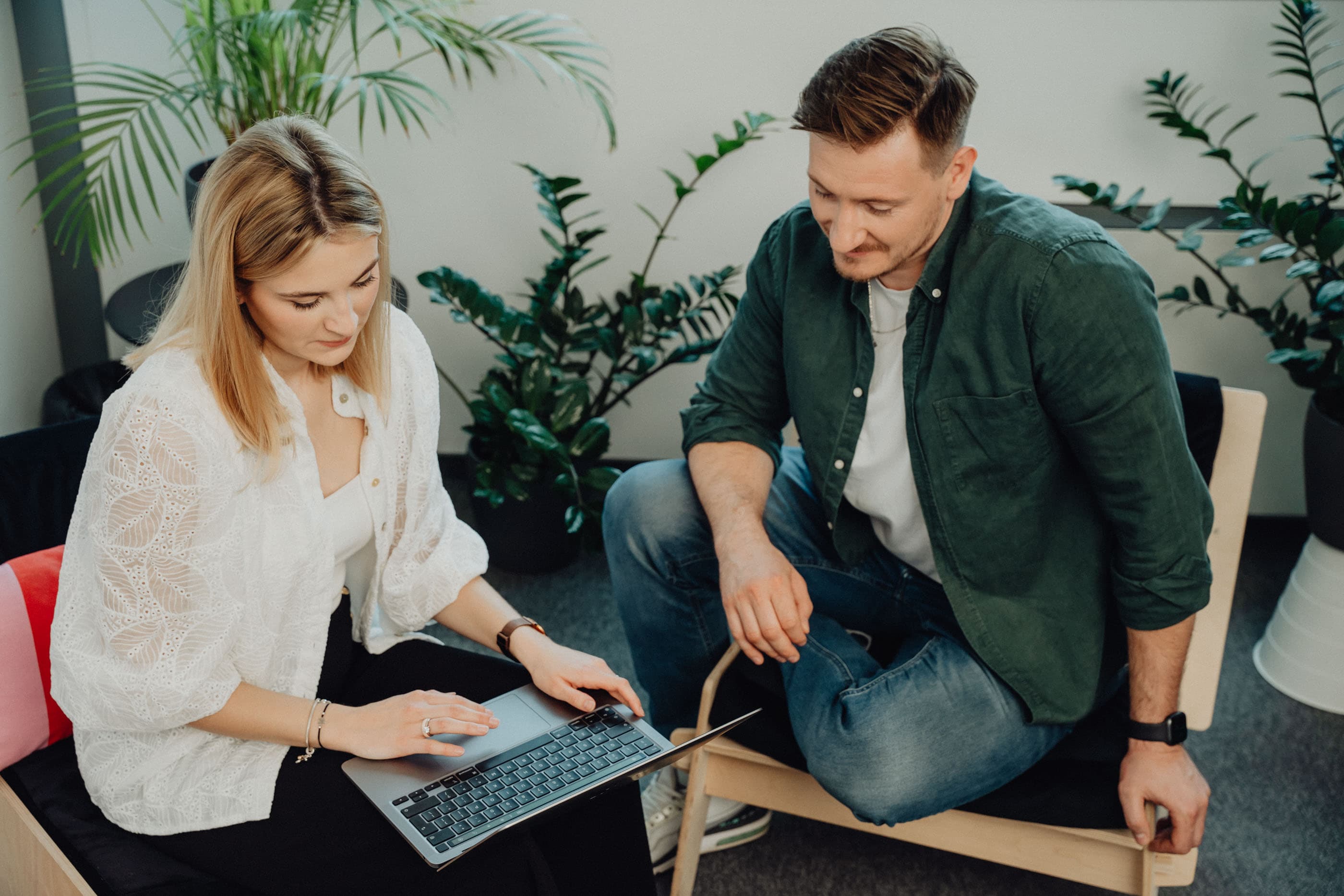
Navigating the Testing Maze: A Clear Guide to QA Automation and Manual Testing
QA automation and manual testing are key to software quality assurance. Discover their benefits, limitations, and how to combine them for optimal results.
Alexander Stasiak
Sep 17, 2024・9 min read
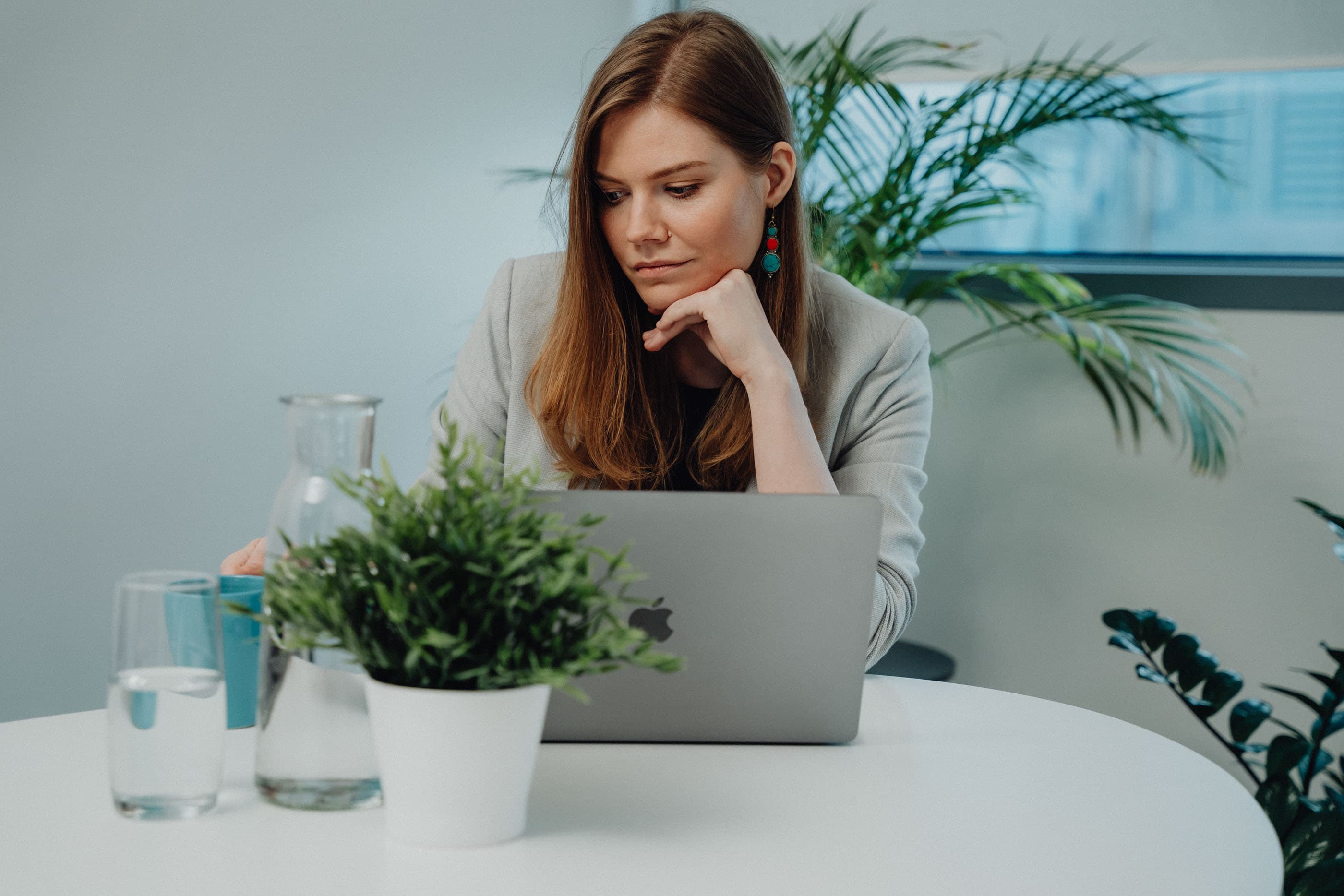
Understanding Acceptance Criteria: A Simple Guide for Beginners
Acceptance criteria are essential for aligning project goals and ensuring quality deliverables. This guide explains their importance, best practices, and role in Agile development.
Alexander Stasiak
Dec 05, 2024・13 min read
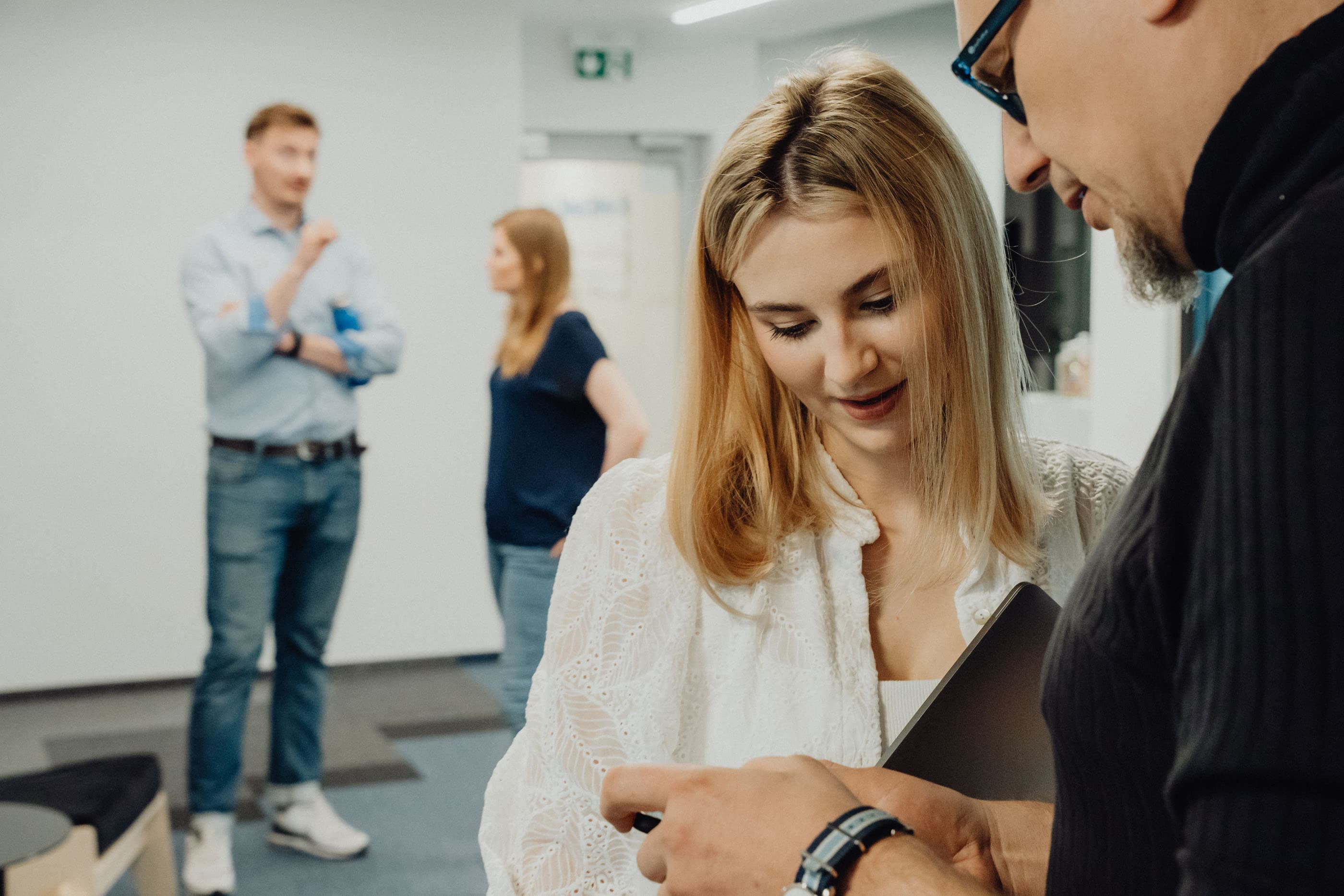
Mastering Quality by Design: A Practical Guide to Success
Discover the core principles of Quality by Design (QbD), a proactive framework ensuring quality in product development. Learn practical strategies for implementation and overcoming challenges.
Alexander Stasiak
Dec 05, 2024・7 min read